AI in Space Industry
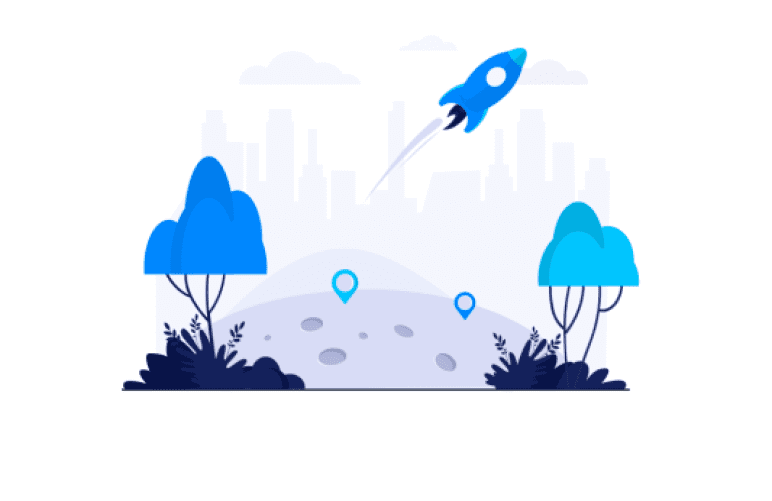
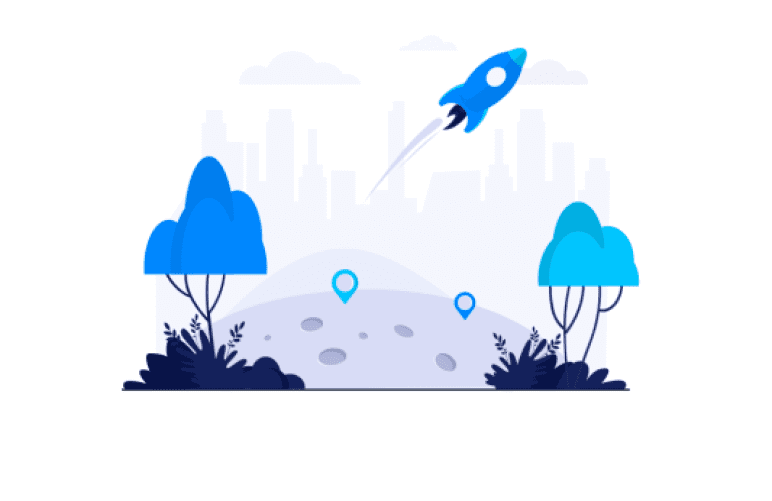
These days, artificial intelligence assumes a focal part in the innovative world and our lives more generally. The developments acquired lately ensure the goal of perplexing issues in unthinkable times.
Artificial intelligence in space exploration is a key piece of the IT system that permits present-day space organizations to develop.
This information should be accessible almost immediately and refreshed continuously by rockets and space stations, which are many kilometers. In summary, the safety of spacecraft and their passengers are guaranteed thanks to extremely powerful machines combined with the latest storage technologies. The demand for technologies expands and information should be focused on and set at the core of plan and advancement.
Most common AI Use Cases
Satellite Object Detection
Handling of satellite information, while utilizing ai in space, helps Earth perception satellites produce a vast amount of information that should be appropriately handled. This information could be retrieved by ground stations and should be gathered and dissected utilizing proper calculations. In this sense, computerized reasoning can ensure the chance of breaking down data in an itemized and proficient manner. For instance, one study can present heat produced in urban areas, other can couple meteorological information with satellite pictures to infer assessments of wind properties, while third can create monitoring systems for the sake of the satellites themselves.
The AI Superior team developed a web application that allows users to communicate with a Custom LLM through a chatbot interface. This innovation empowers organizations to establish private, hosted
A workplace hygiene solutions company approached AI Superior with a unique task: to create a system capable of autonomously identifying when an area needed cleaning, reducing the need for
In today’s dynamic real estate market, accurately assessing the price of different zones within a city is essential for real estate professionals. However, this task has traditionally been challenging
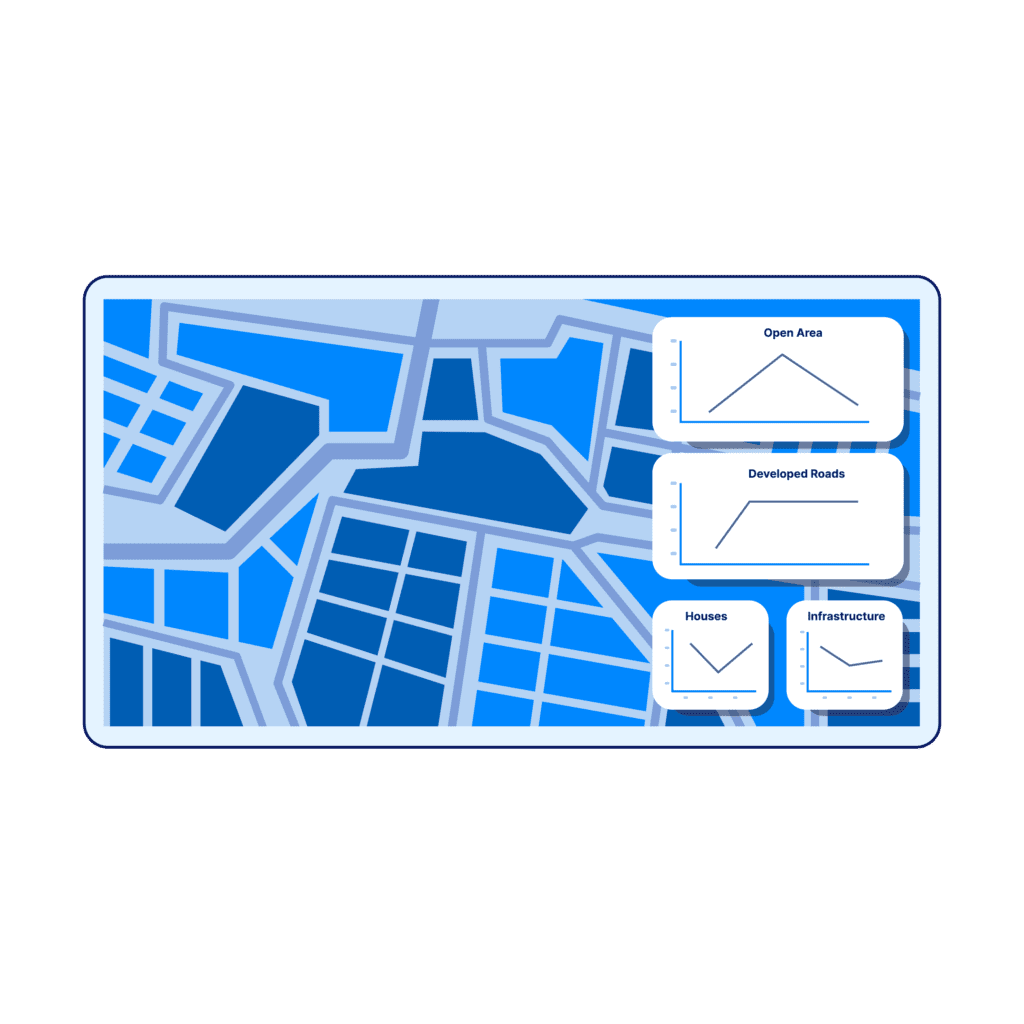
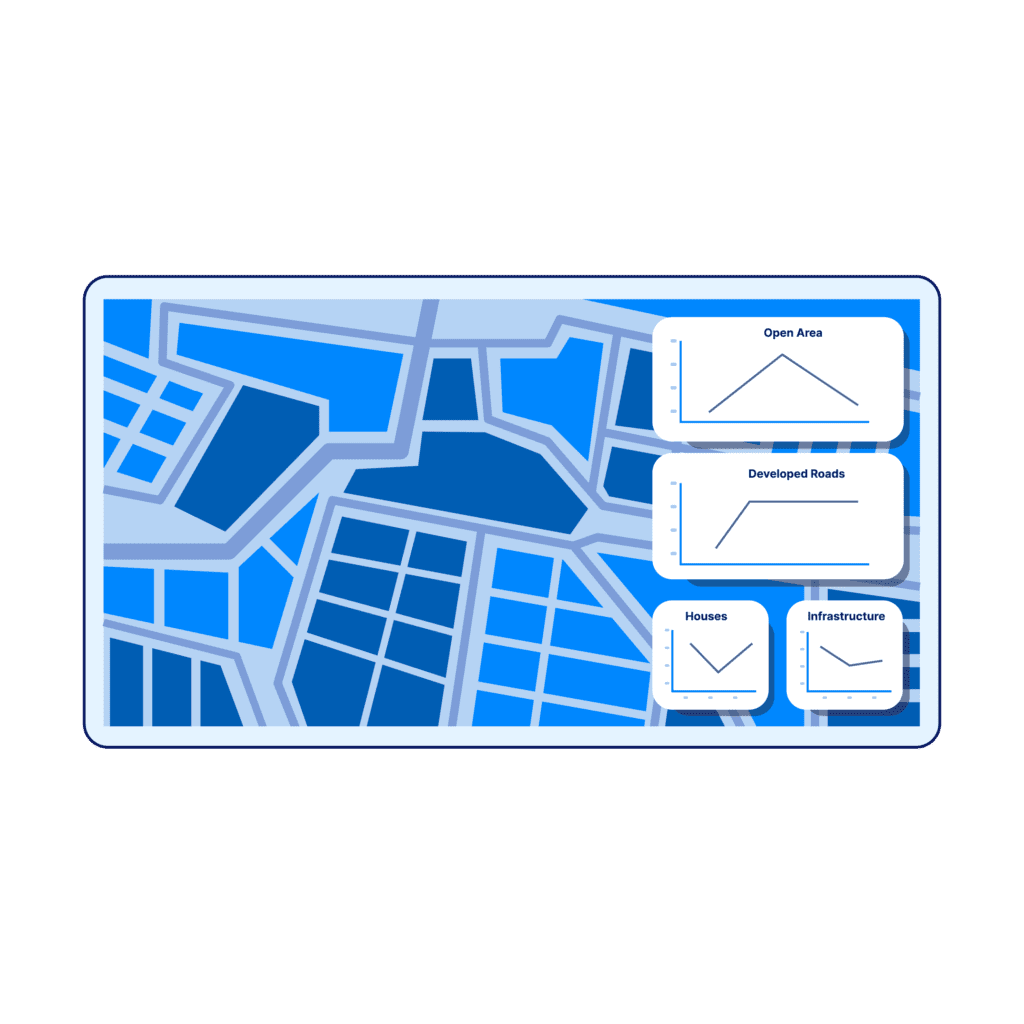
AI Superior, in collaboration with an Ophthalmology Centre, has developed an advanced deep learning model to estimate the volume of fat and muscle in human eyes using CT and
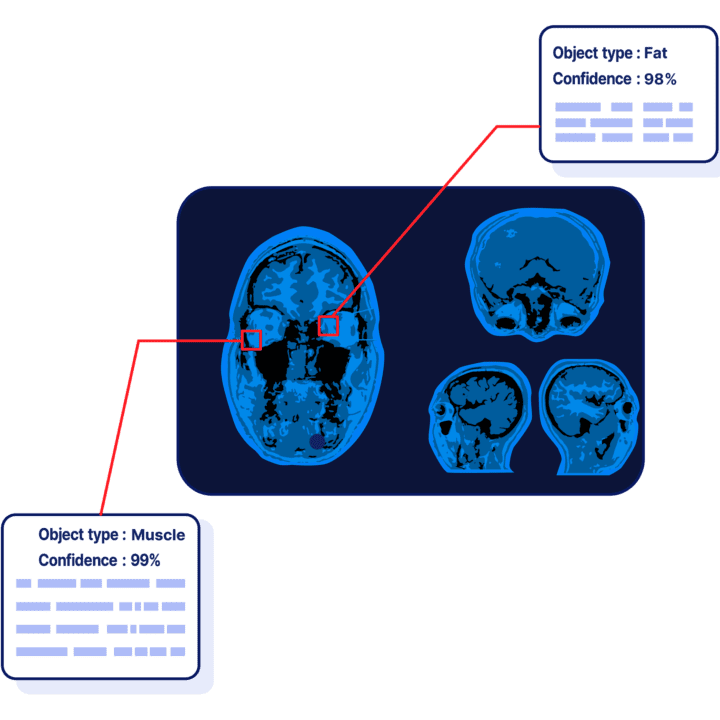
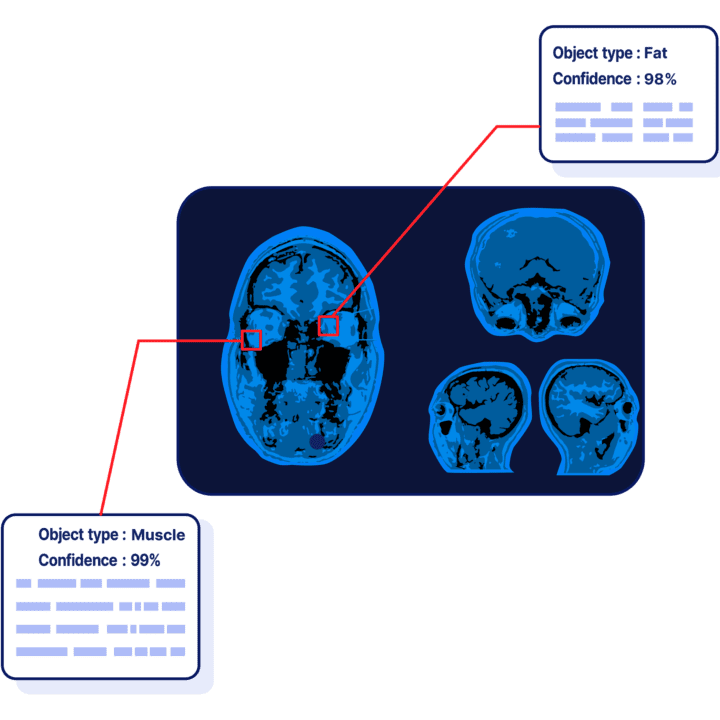
AI Superior has developed an innovative solution for an insurance company that was seeking to provide usage-based insurance to their customers. Leveraging deep learning algorithms, AI Superior has created
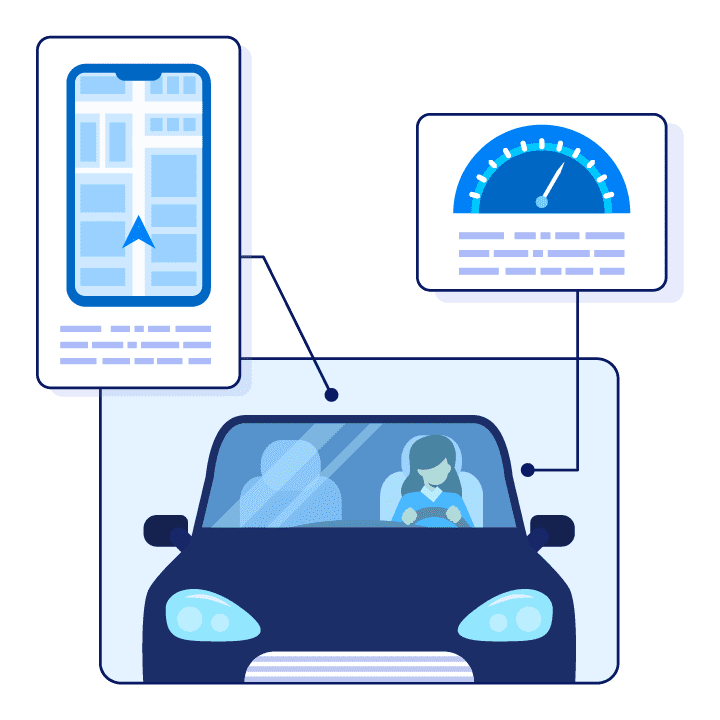
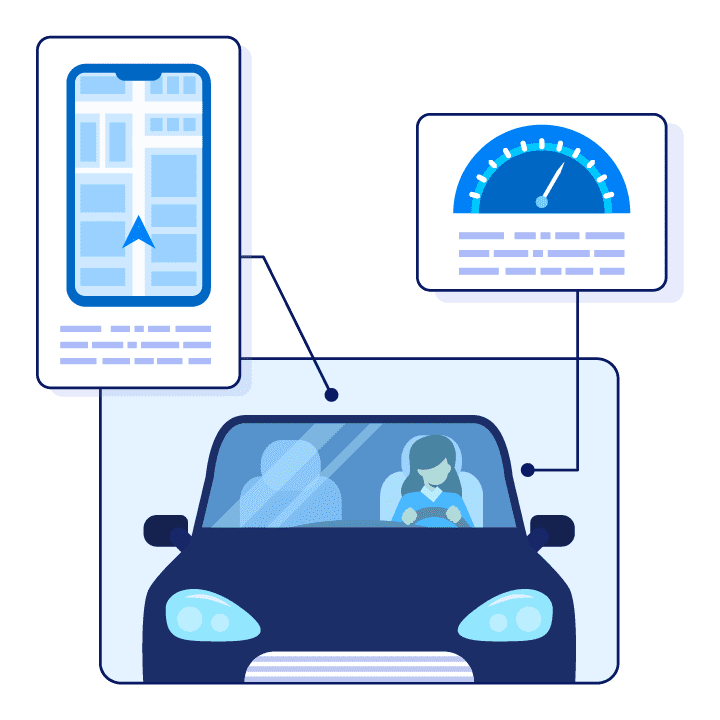
AI Superior has designed an innovative solution for municipalities to rapidly detect and localise graffiti in their cities, using state-of-the-art deep learning algorithms. This real-time, high-accuracy graffiti detection system
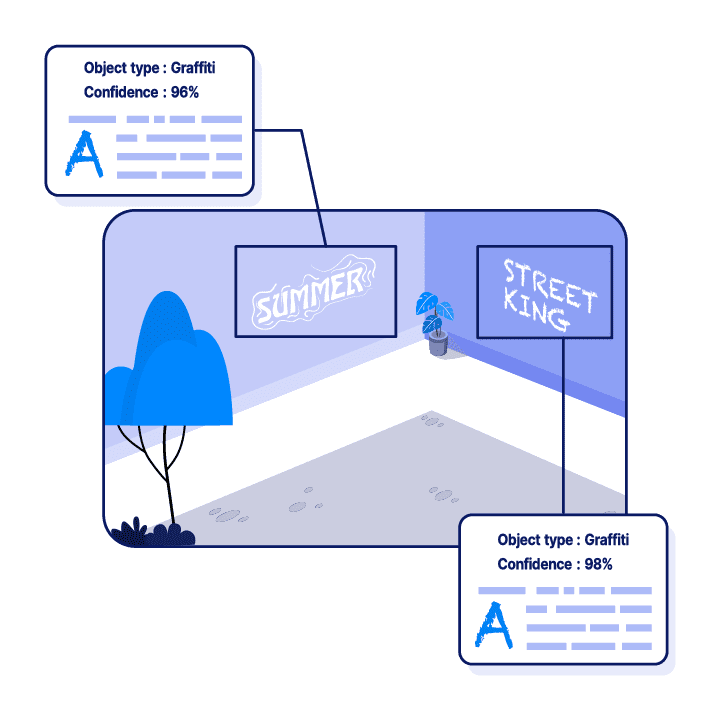
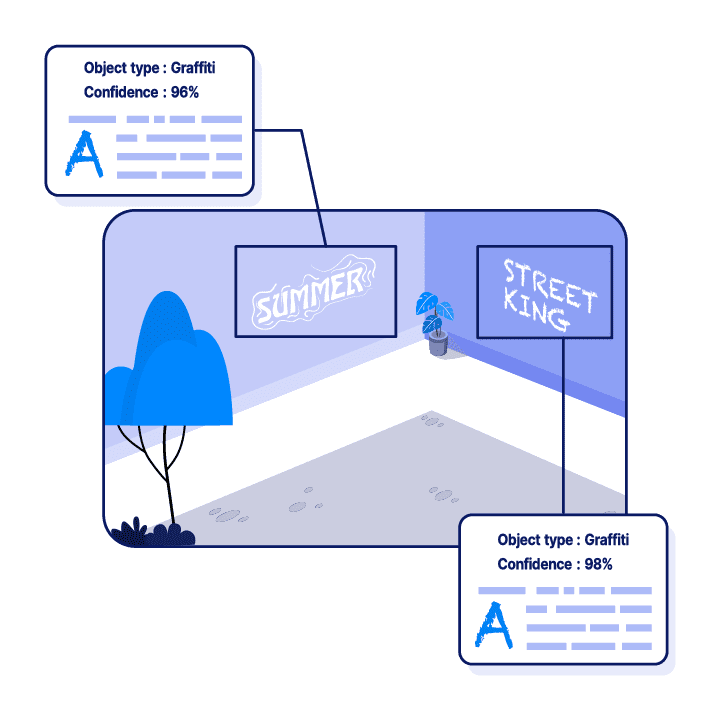
Send Us a Message
By clicking Submit, you agree to our Privacy Policy.